A gentle introduction to algorithmic fairness
Posted on Tue 23 April 2019 in data science
A gentle introduction to issues of algorithmic fairness: some U.S. history, legal motivations, and four definitions with counterarguments.
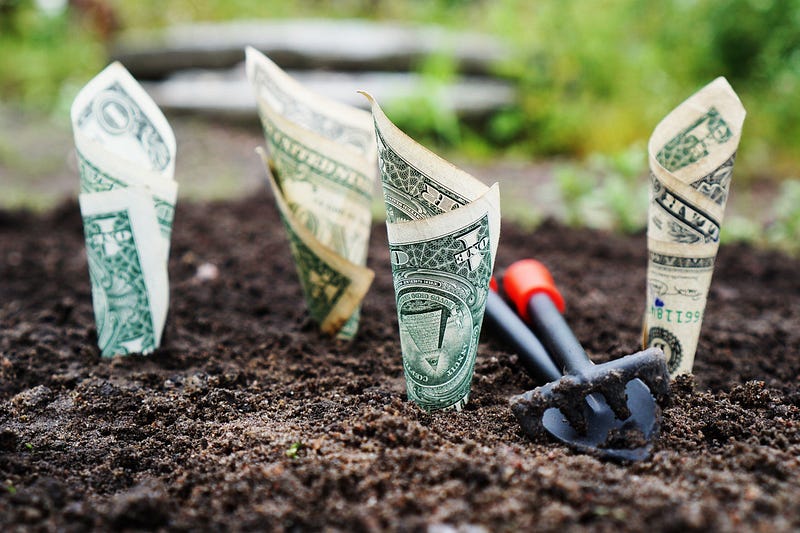
History
In the United States, there is a long history of fairness issues in lending.
For example, redlining:
‘In 1935, the Federal Home Loan Bank Board asked the Home Owners’ Loan Corporation to look at 239 cities and create “residential security maps” to indicate the level of security for real-estate investments in each surveyed city. On the maps, “Type D” neighborhoods were outlined in red and were considered the most risky for mortgage support..
‘In the 1960s, sociologist John McKnight coined the term “redlining” to describe the discriminatory practice of fencing off areas where banks would avoid investments based on community demographics. During the heyday of redlining, the areas most frequently discriminated against were black inner city neighborhoods…’
Redlining is clearly unfair, since the decision to invest was not based on an individual homeowner’s ability to repay the loan, but rather on location; and that basis systematically denied loans to one racial group, black people. In fact, part 1 of a Pulitzer Prize-winning series in the Atlanta Journal-Constitution in 1988 suggests that location was more important than income: “ Among stable neighborhoods of the same income [in metro Atlanta], white neighborhoods always received the most bank loans per 1,000 single-family homes. Integrated neighborhoods always received fewer. Black neighborhoods — including the mayor’s neighborhood — always received the fewest. “
Legislation such as the 1968 Fair Housing Act and the 1977 Community Reinvestment Act were passed to combat these sorts of unfair practices in housing and lending.
More recently, in 2018, WUNC reported that blacks and latinos in some cities in North Carolina were denied mortgages at higher rates than whites:
“Lenders and their trade organizations do not dispute the fact that they turn away people of color at rates far greater than whites. But they maintain that the disparity can be explained by two factors the industry has fought to keep hidden: the prospective borrowers’ credit history and overall debt-to-income ratio. They singled out the three-digit credit score — which banks use to determine whether a borrower is likely to repay a loan — as especially important in lending decisions.”
The WUNC example raises an interesting point: it is possible to look unfair via one measure (loan rates by demographic), but not by another (ability to pay as judged by credit history and debt-to-income ratio). Measuring fairness is complicated. In this case, we can’t tell if the lending practices are fair because the data on credit history and debt-to-income ratio for these particular groups are not available to us to evaluate lenders’ explanations of the disparity.
In 2007, the federal reserve board (FRB) reported on credit scoring and its effects on the availability and affordability of credit. They concluded that the credit characteristics included in credit history scoring models are not a proxy for race, although different demographic groups have substantially different credit scores on average, and “for given credit scores, credit outcomes — including measures of loan performance, availability, and affordability — differ for different demographic groups.” This FRB study supports the lenders’ claims that credit score might explain disparity in mortgage denial rates (since demographic groups have different credit scores), while also pointing out that credit outcomes are different for different groups.
Is this fair or not?
Defining fairness
As machine learning (ML) becomes widespread, there is growing interest in fairness, accountability, and transparency in ML (e.g., the fat* conference and fatml workshops).
Some researchers say that fairness is not a statistical concept, and no statistic will fully capture it. There are many statistical definitions that people try to relate to (if not define) fairness.
First, here are two legal concepts that come up in many discussions on fairness:
- Disparate treatment: “unequal behavior toward someone because of a protected characteristic (e.g., race or gender) under Title VII of the United States Civil Rights Act.” Redlining is disparate treatment if the intent is to deny black people loans.
- Disparate impact: “practices .. that adversely affect one group of people of a protected characteristic more than another, even though rules applied .. are formally neutral.” (“The disparate impact doctrine was formalized in the landmark U.S. Supreme Court case Griggs v. Duke Power Co. (1971). In 1955, the Duke Power Company instituted a policy that mandated employees have a high school diploma to be considered for promotion, which had the effect of drastically limiting the eligibility of black employees. The Court found that this requirement had little relation to job performance, and thus deemed it to have an unjustified — and illegal — disparate impact.” [Corb2018])
[Lipt2017] points out that these are legal concepts of disparity, and creates corresponding terms for technical concepts of parity applied to machine learning classifiers:
- Treatment parity: a classifier should be blind to a given protected characteristic. Also called anti-classification in [Corb2018], or “fairness through unawareness.”
- Impact parity: the fraction of people given a positive decision should be equal across different groups. This is also called demographic parity, statistical parity, or independence of the protected class and the score [Fair2018].
There is a large body of literature on algorithmic fairness. From [Corb2018], two more definitions:
- Classification parity: some given measure of classification error is equal across groups defined by the protected attributes. [Hard2016] called this equal opportunity if the measure is true positive rates, and equalized odds if there were two equalized measures, true positive rates and false positive rates.
- Calibration: outcomes are independent of protected attributes conditional on risk score. That is, reality conforms to risk score. For example, about 20% of all loans predicted to have a 20% chance of default actually do.
There is lack of consensus in the research community on an ideal statistical definition of fairness. In fact, there are impossibility results on achieving multiple fairness notions simultaneously ([Klei2016] [Chou2017]). As we noted previously, some researchers say that fairness is not a statistical concept.
No definition is perfect
Each statistical definition described above has counterarguments.
Treatment parity unfairly ignores real differences. [Corb2018] describes the case of the COMPAS score used to predict recidivism (whether someone will commit a crime if released from jail). After controlling for COMPAS score and other factors, women are less likely to recidivate. Thus, ignoring sex in this prediction might unfairly punish women. Note that the Equal Credit Opportunity Act legally mandates treatment parity: “ Creditors may ask you for [protected class information like race] in certain situations, but they may not use it when deciding whether to give you credit or when setting the terms of your credit. “ Thus, [Corb2018] implies that this sort of unfairness is enshrined in law.
Impact parity doesn’t ensure fairness (people argue against quotas), and can cripple a model’s accuracy, harming the model’s utility to society. [Hard2016] discusses this issue (using the term “demographic parity”) in its introduction.
Corbett et al. [Corb2018] argue at length that classification parity is naturally violated: “ when base rates of violent recidivism differ across groups, the true risk distributions will necessarily differ as well — and this difference persists regardless of which features are used in the prediction. “
They also argue that calibration is not sufficient to prevent unfairness. Their hypothetical example is a bank that gives loans based solely on the default rate within a zip code, ignoring other attributes like income. Suppose that (1) within zip code, white and black applicants have similar default rates; and (2) black applicants live in zip codes with relatively high default rates. Then the bank’s plan would unfairly punish creditworthy black applicants, but still be calibrated.
Conclusion
In summary, likely fairness has no single measure. We took a whirlwind tour of four statistical definitions, two motivated by history and two more recently motivated by machine learning, and summarized the counterarguments to each.
This also means it is challenging to automatically decide if an algorithm is fair. Open-source fairness-measuring packages reflect this by offering many different measures.
However, this doesn’t mean we should ignore statistical measures. They can give us an idea of whether we should look more carefully. Food for thought. We should feed our brain well, it being the most likely to make the final call.
(A note: this subject is rightfully contentious. Our intention is to add to the conversation in a productive, respectful way. We welcome feedback of any kind.)
Thanks to Krishnaram Kenthapadi, Zack Lipton, Luke Merrick, Amit Paka, and Krishna Gade for their feedback.
References
- [Chou2017] Chouldechova, Alexandra. “Fair Prediction with Disparate Impact: A Study of Bias in Recidivism Prediction Instruments.” Big Data 5, no. 2 (June 1, 2017): 153–63. https://doi.org/10.1089/big.2016.0047.
- [Corb2018] Corbett-Davies, Sam, and Sharad Goel. “The Measure and Mismeasure of Fairness: A Critical Review of Fair Machine Learning.” ArXiv:1808.00023 [Cs], July 31, 2018. http://arxiv.org/abs/1808.00023.
- [Fair2018] “Fairness and Machine Learning.” Accessed April 9, 2019. https://fairmlbook.org/.
- [Hard2016] Hardt, Moritz, Eric Price, and Nathan Srebro. “Equality of Opportunity in Supervised Learning.” ArXiv:1610.02413 [Cs], October 7, 2016. http://arxiv.org/abs/1610.02413.
- [Klei2016] Kleinberg, Jon, Sendhil Mullainathan, and Manish Raghavan. “Inherent Trade-Offs in the Fair Determination of Risk Scores.” ArXiv:1609.05807 [Cs, Stat], September 19, 2016. http://arxiv.org/abs/1609.05807.
- [Lipt2017] Lipton, Zachary C., Alexandra Chouldechova, and Julian McAuley. “Does Mitigating ML’s Impact Disparity Require Treatment Disparity?” ArXiv:1711.07076 [Cs, Stat], November 19, 2017. http://arxiv.org/abs/1711.07076.
Originally published at https://blog.fiddler.ai on April 23, 2019.